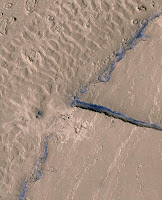
Here is a short example showing the power of Compressed Sensing. I modified a small program written by
Emmanuel Candes from his short course at IMA (
"The role of probability in compressive sampling", Talks(A/V) (ram)).
Before you continue on, you need to have
Matlab and
SeDuMi installed on your system. Let us imagine you have a function f that is unknown to you but for which you know it is sparsely composed of sine functions. Let us imagine for the sake of argument that:
f(x)=2*sin(x)+sin(2x)+21*sin(300x)
The normal way of finding what this decomposition is by computing the scalar product of this function f with sines and cosines with as large as possible of frequency content (in order to capture the high frequency content of this function). This is done iteratively because one doesn't know in advance that a frequency of 300 is part of the solution. But in effect, one is solving a least square problem.
With Compressed Sensing, we know that if we are to measure this function with an incoherent basis to sines and cosines, we are likely to find the decomposition using the L1 minimization. We know that diracs and sines are incoherent (please note we are not using random projections), so we evaluate the scalar product of f with several diracs functions centered at say 18 different locations. In effect, we query for the value of f(x) at the following points x -chosen at random by hand- : 1, 4, 6, 12, 54, 69, 75, 80, 89, 132, 133, 152, 178, 230, 300, 340, 356, 400
We then use these values to solve for the coefficients of the sine series expansion of f(x) by doing an L1 minimization using SeDuMi:
clear
% size of signal
n = 512;
x0 = zeros(n,1);
% function is f(x)=2*sin(x)+sin(2x)+21*sin(300x)
x0(1)=2;
x0(2)=1;
x0(300)=21;
% evaluating f at all sample points xx
% f(1), f(4), f(6).....f(340) f(356) f(400)
xx=[1 4 6 12 54 69 75 80 89 132 133 152 178 230 300 340 356 400];
% C is measurement matrix
for i=1:n
C(:,i)=sin(i*xx)';
end
b = C*x0;
% b is the result of evaluating f at all sample points xx
% f(1), f(4), f(6).....f(340) f(356) f(400)
% C is the measurement matrix
% let us solve for x and see if it is close to x0
% solve l1-minimization using SeDuMi
cvx_begin
variable x(n);
minimize(norm(x,1));
subject to
C*x == b;
cvx_end
figure(1)
plot(abs(x-x0),'o')
title('Error between solution solution found by L1 and actual solution')
figure(2)
plot(x,'*')
hold
plot(x0,'o')
title('Solution found for x using L1 minimization')
With only 20 function evaluations we have a near exact reconstruction. You may want to try the the L2 norm (or least-square) by replacing:
minimize(norm(x,1));
by
minimize(norm(x,2));
and then change the number of function evaluations needed to reach the same result.
With 200 functions evaluations, the error between the reconstructed solution and the actual solution is several orders of magnitude larger than the L1 technique with 20 functions evaluations.
Pretty neat uh ?
Liked this entry ? subscribe to the Nuit Blanche feed, there's more where that came from
If you think this blog provides a service, please support it by ordering through the
Amazon - Nuit Blanche Reference Store